Development, validation and application of in-silico methods to predict the macromolecular targets of small organic compounds
Doctoral thesis
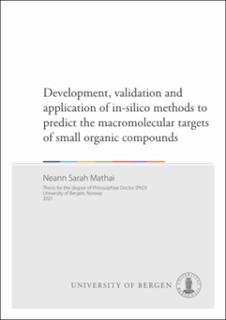
Åpne
Permanent lenke
https://hdl.handle.net/11250/3001272Utgivelsesdato
2021-12-10Metadata
Vis full innførselSamlinger
- Department of Chemistry [433]
Sammendrag
Computational methods to predict the macromolecular targets of small organic drugs and drug-like compounds play a key role in early drug discovery and drug repurposing efforts. These methods are developed by building predictive models that aim to learn the relationships between compounds and their targets in order to predict the bioactivity of the compounds.
In this thesis, we analyzed the strategies used to validate target prediction approaches and how current strategies leave crucial questions about performance unanswered. Namely, how does an approach perform on a compound of interest, with its structural specificities, as opposed to the average query compound in the test data? We constructed and present new guidelines on validation strategies to address these short-comings. We then present the development and validation of two ligand-based target prediction approaches: a similarity-based approach and a binary relevance random forest (machine learning) based approach, which have a wide coverage of the target space. Importantly, we applied a new validation protocol to benchmark the performance of these approaches. The approaches were tested under three scenarios: a standard testing scenario with external data, a standard time-split scenario, and a close-to-real-world test scenario. We disaggregated the performance based on the distance of the testing data to the reference knowledge base, giving a more nuanced view of the performance of the approaches. We showed that, surprisingly, the similarity-based approach generally performed better than the machine learning based approach under all testing scenarios, while also having a target coverage which was twice as large.
After validating two target prediction approaches, we present our work on a large-scale application of computational target prediction to curate optimized compound libraries. While screening large collections of compounds against biological targets is key to identifying new bioactivities, it is resource intensive and challenging. Small to medium-sized libraries, that have been optimized to have a higher chance of producing a true hit on an arbitrary target of interest are therefore valuable. We curated libraries of readily purchasable compounds by: i. utilizing property filters to ensure that the compounds have key physicochemical properties and are not overly reactive, ii. applying a similaritybased target prediction method, with a wide target scope, to predict the bioactivities of compounds, and iii. employing a genetic algorithm to select compounds for the library to maximize the biological diversity in the predicted bioactivities. These enriched small to medium-sized compound libraries provide valuable tool compounds to support early drug development and target identification efforts, and have been made available to the community.
The distinctive contributions of this thesis include the development and benchmarking of two ligand-based target prediction approaches under novel validation scenarios, and the application of target prediction to enrich screening libraries with biologically diverse bioactive compounds. We hope that the insights presented in this thesis will help push data driven drug discovery forward.
Består av
Paper 1: Mathai, N.; Chen, Y.; Kirchmair, J. Validation strategies for target prediction methods, Briefings in Bioinformatics, 2020, 21(3), pp. 791-802. The article is available at: https://hdl.handle.net/1956/21871Paper 2: Mathai, N.; Kirchmair, J. Similarity-based methods and machine learning approaches for target prediction in early drug discovery: performance and scope, International Journal of Molecular Sciences, 2020, 21(10), 3585. The article is available at: https://hdl.handle.net/11250/2730409
Paper 3: Mathai, N.; Stork, C.; Kirchmair, J. BonMOLière: Small-sized libraries of readily purchasable compounds, optimized to produce genuine hits in biological screens across the protein space, International Journal of Molecular Sciences, 2021, 22(15), 7773. The article is available at: https://hdl.handle.net/11250/2768773