Deep learning-enabled Inference of 3D molecular absorption distribution of biological cells from IR spectra
Magnussen, Eirik Almklov; Zimmermann, Boris; Blazhko, Uladzislau; Dzurendová, Simona; Dupuy--Galet, Benjamin Xavier; Byrtusova, Dana; Muthreich, Florian; Tafintseva, Valeria; Liland, Kristian Hovde; Tøndel, Kristin; Shapaval, Volha; Kohler, Achim
Journal article, Peer reviewed
Published version
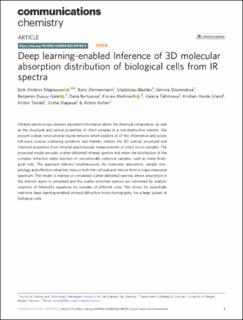
Åpne
Permanent lenke
https://hdl.handle.net/11250/3049682Utgivelsesdato
2022Metadata
Vis full innførselSamlinger
- Department of Biological Sciences [2216]
- Registrations from Cristin [9489]
Sammendrag
Infrared spectroscopy delivers abundant information about the chemical composition, as well as the structural and optical properties of intact samples in a non-destructive manner. We present a deep convolutional neural network which exploits all of this information and solves full-wave inverse scattering problems and thereby obtains the 3D optical, structural and chemical properties from infrared spectroscopic measurements of intact micro-samples. The proposed model encodes scatter-distorted infrared spectra and infers the distribution of the complex refractive index function of concentrically spherical samples, such as many biological cells. The approach delivers simultaneously the molecular absorption, sample morphology and effective refractive index in both the cell wall and interior from a single measured spectrum. The model is trained on simulated scatter-distorted spectra, where absorption in the distinct layers is simulated and the scatter-distorted spectra are estimated by analytic solutions of Maxwell’s equations for samples of different sizes. This allows for essentially real-time deep learning-enabled infrared diffraction micro-tomography, for a large subset of biological cells.