HALE, the Hip Arthroplasty Longevity Estimation system
Master thesis
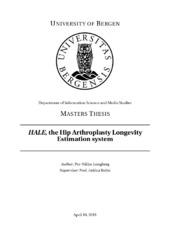
Åpne
Permanent lenke
http://hdl.handle.net/1956/18783Utgivelsesdato
2018-12-15Metadata
Vis full innførselSamlinger
Sammendrag
This master thesis presents a Design Science research in which the HALE system for total hip arthroplasty prosthesis longevity estimation has been developed. The HALE system was developed to explore the use of machine learning techniques on a biomedical dataset motivated by two user groups’ needs - biomedical engineers who analyze explanted hip arthroplasty prostheses and physicians who work with patients and want to know what the safe and optimal treatment for each patient is. The dataset mainly contains biochemical measurements and has a limited number of patient data (demographics). The machine learning techniques are seen as a possibility to quickly and promptly analyze the data and answer questions about specific cases as well as the patient group as a whole. The machine learning components rely on regression analysis for prediction and estimating the outcome of single patient cases, as well as the group. Two methods were implemented - multiple linear regression and an optimized C&RT decision tree. At this point in development users found multiple linear regression more appealing for its transparency and better performance in comparison to the regression based decision tree counterpart. In the future C&RT trees can be considered as an alternative when the users have more experience and trust rely on the system. The machine learning methods used in the HALE system were validated against a comparative linear regression statistical procedure of IBMs SPSS software, resulting in a comparable accuracy, performance and similarly constructed regression model. User evaluation has shown that the HALE system was manageable and appealing to the user groups. The largest current practical limitation is the size of the dataset, however by expanding this dataset and adding new clinical variables it will be easy to improve the performance of the regression models. It is also expected that additional functionality such as discriminant and clustering analysis would be feasible to implement. Thus, the machine learning components of the HALE system, as implemented using scikit-learn, have proven to be suitable and easy to utilize even for novice developers.