Identifying the nature of the QCD transition in heavy-ion collisions with deep learning
Du, Yilun; Zhou, Kai; Steinheimer, Jan; Pang, Long-Gang; Motornenko, Anton; Zong, Hong-Shi; Wang, Xin-Nian; Stöcker, Horst
Journal article, Peer reviewed
Published version
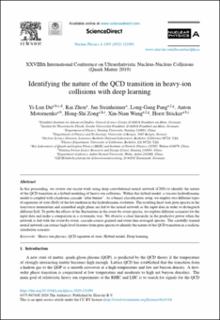
Åpne
Permanent lenke
https://hdl.handle.net/11250/2984929Utgivelsesdato
2021Metadata
Vis full innførselSamlinger
Sammendrag
In this proceeding, we review our recent work using deep convolutional neural network (CNN) to identify the nature of the QCD transition in a hybrid modeling of heavy-ion collisions. Within this hybrid model, a viscous hydrodynamic model is coupled with a hadronic cascade “after-burner”. As a binary classification setup, we employ two different types of equations of state (EoS) of the hot medium in the hydrodynamic evolution. The resulting final-state pion spectra in the transverse momentum and azimuthal angle plane are fed to the neural network as the input data in order to distinguish different EoS. To probe the effects of the fluctuations in the event-by-event spectra, we explore different scenarios for the input data and make a comparison in a systematic way. We observe a clear hierarchy in the predictive power when the network is fed with the event-by-event, cascade-coarse-grained and event-fine-averaged spectra. The carefully-trained neural network can extract high-level features from pion spectra to identify the nature of the QCD transition in a realistic simulation scenario.