Measuring Adversarial Robustness using a Voronoi-Epsilon Adversary
Journal article, Peer reviewed
Published version
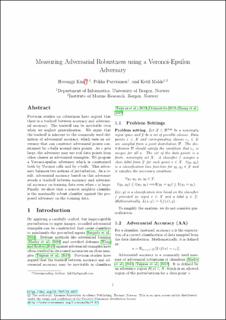
Åpne
Permanent lenke
https://hdl.handle.net/11250/3123382Utgivelsesdato
2023Metadata
Vis full innførselSamlinger
- Department of Informatics [917]
- Registrations from Cristin [9489]
Sammendrag
Previous studies on robustness have argued that there is a tradeoff between accuracy and adversarial accuracy. The tradeoff can be inevitable even when we neglect generalization. We argue that the tradeoff is inherent to the commonly used definition of adversarial accuracy, which uses an adversary that can construct adversarial points constrained by $\epsilon$-balls around data points. As $\epsilon$ gets large, the adversary may use real data points from other classes as adversarial examples. We propose a Voronoi-epsilon adversary which is constrained both by Voronoi cells and by $\epsilon$-balls. This adversary balances two notions of perturbation. As a result, adversarial accuracy based on this adversary avoids a tradeoff between accuracy and adversarial accuracy on training data even when $\epsilon$ is large. Finally, we show that a nearest neighbor classifier is the maximally robust classifier against the proposed adversary on the training data.